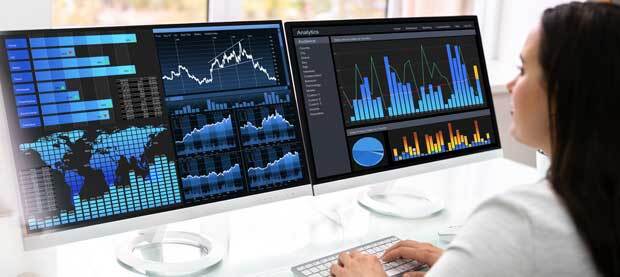
As more leaders prioritize technologies such as machine learning (ML) and big data, they increasingly turn to predictive analytics for decision-making. By developing predictions, organizations are better able to gain a competitive advantage and use resources effectively. In this article, we’ll explore how predictive analytics works across industries, the various models used and the reasons why so many corporations invest heavily in predictive analytics.
What Is Predictive Analytics?
Predictive analytics is a decision-making tool used to predict possible events and determine a plan of action. It uses historical data, statistics, machine learning and analysis to create a model that shows trends or patterns. Analysts input current data to forecast the odds of a future event happening.
Finding and making decisions based on patterns isn’t new, but the introduction of the first computational models in the 1940s signaled a turning point. Indeed, through the years, relational databases, faster central processing units (CPUs) and programming languages made it easier to digest big data.1
According to IBM, “Predictive modeling capabilities are scientifically proven and have benefited from decades of advancement.”2 The global market for predictive analytics is projected to reach approximately $10.95 billion by 2022.3 With its widespread uses across industries, this is a science worth learning and tapping into.
How Predictive Analytics Works
Predictive analytics leverages many different technologies and methods, such as machine learning, mathematical processes, data mining, statistical algorithms and big data.4 It is most effective when using large, dependable data sets and statistical modeling alongside assumptions.5
Organizations can sort through real-time and historical data using these tools and processes. In doing so, they can uncover patterns or trends and develop parameters to forecast future events. By selecting suitable models, companies can learn how various factors relate to each other and use the information to evaluate strategies to reach goals or avoid risks.4
Benefits of Predictive Analytics
With the wealth of available data, any organization can harness it and turn it into actionable insights that offer a competitive edge. Furthermore, the benefits extend to all industries and all departments. Companies that invest in predictive analytics realize many benefits, which include:
- Discovering opportunities for new products or services
- Understanding business customers’ behavior and reasoning
- Reducing operational expenses
- Decreasing business risks
- Foreseeing potential problems
- Optimizing workforce planning
- Improving customer retention
- Detecting fraud
- Staying one step ahead of the competition
- Improving maintenance scheduling
- Increasing equipment and fleet lifespans
Types of Predictive Analytics Models
To answer the question of ‘what could happen in the future,’ predictive analytics uses models. There are many to choose from, and it’s essential to select the right one for your needs. Think about what questions you want to answer and what you want to do with the information. Then consider the strengths of individual models and how they work best with predictive analytics algorithms.6
Analysts use an iterative process to build a model with a training data set. Next, they test and validate the model to assess accuracy. It may take several machine learning approaches to identify the most useful model.7 Predictive analytics models include these:.
Regression Analysis Techniques
Regression analysis examines the “relation between the target or dependent variable and independent variable in a dataset.”8 It is essential to handling regression issues in machine learning using data modeling. To select the right model, analysts consider the shape of the regression line, the kind of target variable and the number of independent variables.8 Regression techniques include:
- Linear regression
- Analysis of variance (ANOVA)
- Ridge regression
- Logistic regression
- Time-series regression
- Polynomial regression
- Lasso regression
- Bayesian linear regression
Classification Analysis
The classification technique categorizes data using binary, multi-class or multi-label classification.9 This data analysis task uses mathematical methods to select and categorize information and improves the accuracy of data analysis. There are many types of classification analysis models, such as:
- Decision trees
- Naive bayes
- Neural networks
- Random forest
- K nearest neighbors
- Support vector machine (SVM)
Clustering Models
For clustering models, analysts separate data into groups according to shared characteristics. Groupings must identify similarities yet show distinct differences from other collections.10 The uses across industries are vast. For instance, business leaders use cluster-based plagiarism detection methods in academics,11 and health organizations find that "clustering works well for 10 out the 14 cancer types."12 The various models consist of:
- Hierarchical clustering
- Distribution-based clustering
- Partitioning methods
- Fuzzy clustering
- Density-based clustering
- Supervised clustering
Forecast Models
Forecast models are commonly used in business applications to develop economic, technological or demand forecasts.13 The techniques used depend on the industry and goals. The kinds of forecast methods include:
- Naive
- Qualitative
- Quantitative
- Casual
- Judgmental
- Time series
How Industries Use Predictive Analytics
From manufacturers developing insights from master data logs to financial professionals assessing creditworthiness, predictive analytics plays a role in all organizations. It helps companies turn data sets into insights used for decision-making. In these sectors, advancements in predictive analytics allow leaders to take a proactive approach:
Healthcare
The internet of things (IoT) provides data used to identify and prioritize patient populations based on risk levels for certain conditions.
Manufacturing
Predictive analytic models using equipment sensor data assist with creating maintenance schedules that increase uptime and prevent costly breakdowns.
Finance
Professionals can leverage models to assess the risks of extending credit to companies or individuals using various individual or group classifications.
Insurance
Predictive analytics helps agencies prevent and detect insurance fraud by tracking potential scammers and cross-referencing in-house information with public data.
Software as a Service (SaaS)
Understanding why and when customers churn helps marketers proactively engage users before those moments occur.
Food and Beverage
Keeping tabs on equipment and products via sensors helps processors and shippers increase operational efficiencies and proactively maintain equipment for consistent food and beverage quality.
Retail
Mining transaction data helps retailers stock shelves with the correct items before big events and recommend products to shoppers based on historical buying habits.
Entertainment
User information helps shape experiences. Streaming platforms use modeling for content suggestions and to come up with new movies predicted to be blockbusters.
Accelerate Your Career by Mastering Predictive Analytics
Tap into the power of predictive analytics to expand your job opportunities and increase your worth. The Online Master of Science in Business Analytics (MSBA) from “Public Ivy” William & Mary can help you master business analytics and unlock the insights of big data.Learn more about the hands-on capstone course with which the Online MSBA culminates and consider how the skills you’ll showcase in completing it can transform your career.
- Retrieved on May 18, 2021 from saleschoice.com/the-history-and-roots-of-predictive-analytics/
- Retrieved on May 18, 2021 from ibm.com/downloads/cas/LKMPR8AJ
- Retrieved on May 18, 2021 from globenewswire.com/news-release/2018/03/02/1414176/0/en/Trends-in-Predictive-Analytics-Market-Size-Share-will-Reach-10-95-Billion-by-2022.html
- Retrieved on May 18, 2021 from cio.com/article/3273114/what-is-predictive-analytics-transforming-data-into-future-insights.html
- Retrieved on May 18, 2021, from, verypossible.com/insights/what-is-predictive-analytics
- Retrieved on May 18, 2021 from logianalytics.com/predictive-analytics/predictive-algorithms-and-models/
- Retrieved on May 18, 2021 from mathworks.com/discovery/predictive-analytics.html
- Retrieved on May 18, 2021 from upgrad.com/blog/types-of-regression-models-in-machine-learning/
- Retrieved on May 18, 2021 from analyticsindiamag.com/7-types-classification-algorithms/
- Retrieved on May 18, 2021 from analytixlabs.co.in/blog/types-of-clustering-algorithms/
- Retrieved on May 18, 2021 from pan.webis.de/downloads/publications/papers/du_2010.pdf
- Retrieved on May 18, 2021 from ncbi.nlm.nih.gov/pmc/articles/PMC5634820/
- Retrieved on May 18, 2021 from mech.at.ua/Forecasting.pdf